Welcome to ISMG's Ransomware Resource Center!
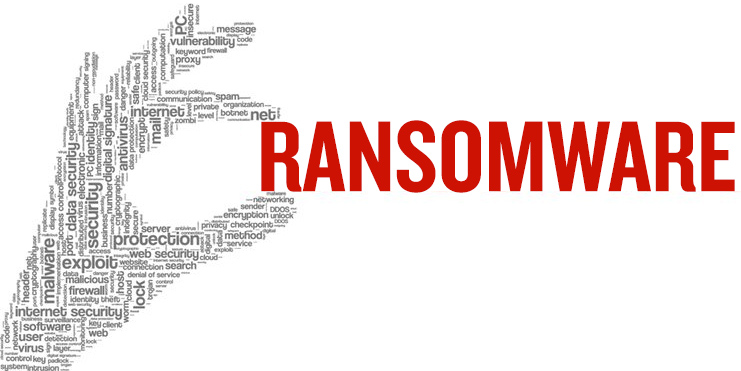
A suspected initial access specialist for a ransomware-wielding group is being extradited from Ukraine to the United States to stand trial. The group has been accused of earning over $100 million in ransom by using malware such as Ryuk, Dharma and Hive against more than 2,400 organizations.